The Four Pillars of Data Observability
Discover the core pillars of data observability and how they provide the essential insights needed to monitor, manage, and improve data health.
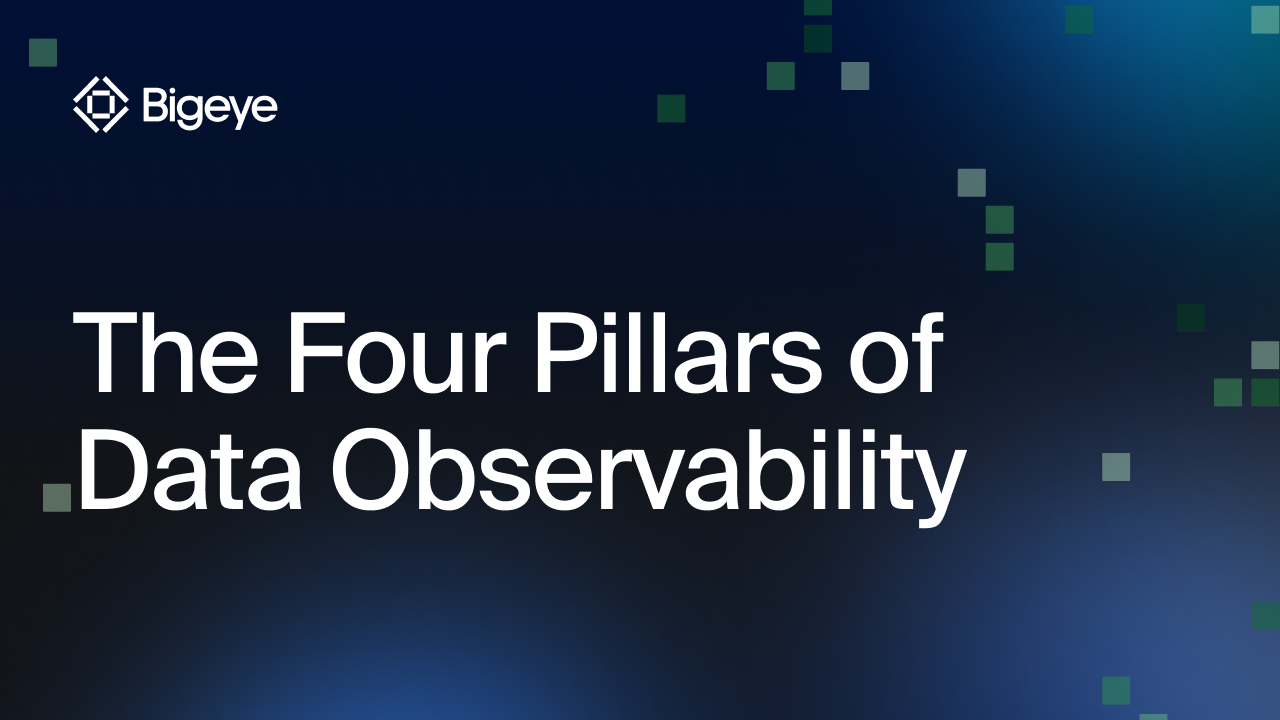
Get the Best of Data Leadership
Stay Informed
Get Data Insights Delivered
Data observability has become a critical part of ensuring modern data systems are reliable, accurate, and performing as expected. As data environments grow more complex, the ability to effectively monitor, understand, and manage them is no longer optional—it's essential. At the core of data observability are four key pillars: metrics, metadata, lineage, and logs. This article dives into each pillar to explore their individual roles and how they come together to strengthen data observability.
The Purpose of the Four Pillars of Data Observability
The four pillars of data observability—metrics, metadata, lineage, and logs—serve as the foundational elements that provide comprehensive visibility into data systems. These pillars work together to offer insights into the state and behavior of data as it moves through various stages of processing. By focusing on these four aspects, data observability allows organizations to detect and diagnose issues, monitor data health, and ensure the reliability of data pipelines.
The purpose of these pillars is to provide a holistic view of data environments, enabling proactive management and troubleshooting. They help data teams to understand the flow and transformations of data, detect anomalies, and trace issues to their root causes. This comprehensive understanding is critical for maintaining data quality, ensuring compliance with regulations, and supporting data-driven decision-making.
Software Observability vs. Data Observability
Before diving into the pillars of data observability, it's important to distinguish between software observability and data observability. While they share similar concepts, their focus areas differ.
Software observability involves monitoring and understanding the state of software systems and applications. It uses metrics, logs, and traces to provide insights into the performance and behavior of software, helping teams identify and resolve issues in real time. The focus is on the software's operational health, ensuring that applications run smoothly and efficiently.
Data observability, on the other hand, focuses specifically on the data flowing through various systems. While it also uses metrics, logs, and other tools, the goal is to understand the state of the data itself—its quality, transformations, movement, and usage. Data observability aims to ensure that data is reliable, accurate, and available for use in decision-making processes.
Both types of observability are essential in modern IT environments, but they address different aspects of system health. Software observability ensures the operational performance of applications, while data observability ensures the integrity and reliability of the data those applications depend on.
Metrics
Metrics are a critical component of data observability. They provide quantifiable data points that offer insights into the performance and health of data systems. In the context of data observability, metrics can include a wide range of indicators, such as data freshness, completeness, and accuracy.
Data Freshness
This metric measures how up-to-date the data is. It indicates whether data is being processed and delivered within expected time frames, ensuring that it is timely and relevant.
Data Completeness
Completeness metrics assess whether all expected data is present. Missing or incomplete data can lead to inaccurate analyses and decisions.
Data Accuracy
Accuracy metrics evaluate how closely the data reflects the real-world entities or events it represents. Inaccurate data can cause significant downstream issues.
Metrics are used to monitor the performance of data pipelines and systems continuously. By tracking these metrics over time, organizations can identify trends, detect anomalies, and take corrective actions before issues impact business operations. We have previously written more extensively on data quality metrics so make sure you check out that post.
Metadata
Metadata is another crucial pillar of data observability. It provides descriptive information about data, including its origin, structure, format, and the transformations it has undergone. Metadata is essential for understanding the context of data and ensuring that it can be interpreted and used correctly.
In data observability, metadata serves several key functions.
Data Context
Metadata provides context for data, helping users understand its meaning, origin, and usage. This context is critical for accurate data analysis and decision-making.
Tracking Transformations
Metadata captures information about the transformations and processes that data has undergone. This helps in tracing the journey of data through various stages of processing, ensuring that all changes are documented and understood.
Governance and Compliance
Metadata plays a key role in data governance, helping organizations enforce data policies and comply with regulations. It ensures that data is used in accordance with established standards and legal requirements.
By maintaining comprehensive metadata, organizations can ensure that their data is well-documented, easily discoverable, and properly governed. This supports data observability by providing the necessary context for monitoring and managing data systems.
Lineage
Data lineage is the third pillar of data observability. It involves tracing the path of data as it moves through different systems, processes, and stages of a data pipeline. Lineage provides a detailed map of where data comes from, how it is transformed, and where it ends up.
Data Flow Tracking
Data lineage tracks the flow of data from its source to its final destination. This includes all the transformations, aggregations, and enrichments that data undergoes along the way.
Impact Analysis
By understanding data lineage, organizations can assess the impact of changes in data sources, transformations, or destinations. This is critical for managing the effects of updates, ensuring that downstream processes are not adversely affected.
Error Tracing
When issues arise, data lineage allows teams to trace the problem back to its source. This capability is essential for diagnosing and resolving errors quickly, minimizing downtime and ensuring data integrity.
Data lineage is essential for understanding the dependencies within a data ecosystem. It provides visibility into how data moves and changes, enabling organizations to manage complexity and ensure the accuracy of their data.
Logs
Logs are the fourth pillar of data observability. They provide detailed records of events that occur within data systems, capturing information about operations, errors, and other activities. Logs are critical for troubleshooting and understanding the behavior of data systems.
Event Tracking
Logs capture detailed information about events within data systems, such as data ingestion, processing steps, and errors. This information is essential for understanding what happens at each stage of a data pipeline.
Error Diagnosis
When issues occur, logs provide the information needed to diagnose and resolve the problem. They offer a chronological record of events, helping teams identify where and when an issue arose.
Audit Trails
Logs serve as audit trails, documenting the activities that occur within data systems. This is important for compliance and governance, as it provides a record of how data is handled and processed.
Logs are a fundamental tool for maintaining data observability. They provide the granular detail needed to monitor data systems, diagnose issues, and ensure that data processes are running smoothly.
Bringing It All Together
The four pillars of data observability—metrics, metadata, lineage, and logs—are not standalone elements. They work together to provide a comprehensive view of data systems, enabling organizations to monitor, manage, and optimize their data environments effectively. Here are a few examples:
- Integrating Metrics and Logs: Metrics provide high-level insights into the performance of data systems, while logs offer detailed event records. Together, they enable real-time monitoring and troubleshooting, allowing teams to detect issues early and resolve them quickly.
- Using Metadata for Context: Metadata provides the context needed to interpret metrics and logs accurately. By understanding the structure, origin, and transformations of data, teams can make informed decisions and ensure that data is used correctly.
- Leveraging Lineage for Impact Analysis: Data lineage allows organizations to understand the dependencies within their data ecosystems. This is essential for managing changes, ensuring that updates do not disrupt downstream processes, and maintaining data integrity.
- Establishing a Feedback Loop: The pillars of data observability should be integrated into a continuous feedback loop. Metrics, logs, metadata, and lineage should be monitored and analyzed regularly to identify trends, detect anomalies, and improve data processes over time.
By bringing these four pillars together, you gain the clarity and confidence needed to effectively manage your data environments and maintain data health at scale.
Ready to take control of your data systems with comprehensive observability? Discover how Bigeye’s platform leverages the four pillars to ensure your data is reliable, accurate, and always available. Schedule a demo today to see how we can help you manage your data with confidence.
Monitoring
Schema change detection
Lineage monitoring