Getting started with dbt tests
Increase data quality by validating your dbt transformations.
.webp)
When running data build tools (dbt), you might want to perform simple validations. Perhaps it's worth checking that a source table has successfully transformed into a model, or that the source table doesn’t contain too many nulls.
One great way to validate is through testing. Testing is a supported feature in dbt, where you create test cases for your transformations. Through tests, you catch potential issues earlier, saving you the effort of fixing the issue downstream.
In this guide, you will test an ELT pipeline project based on publicly available COVID-19 data from Google BigQuery Public Datasets Program. We’ll cover some aspects of creating and running dbt tests on the transformations. We'll cover:
- Overview of testing in dbt
- dbt core built-in tests
- dbt custom tests
Setup
- Create a Snowflake warehouse (you get up to $400 in credits without a credit card)
- Extract and load COVID-19 data from Google BigQuery Public Datasets Program
- using Airbyte into the Snowflake warehouse
- Install DBT Core/DBT CLI
- Clone this Github repo as your dbt project
- Connect DBT Core to your Snowflake Warehouse by creating a profiles.yml in your dbt project and configuring it with your Snowflake warehouse information. You can copy the
- sample_profile.yml file currently in the repo.
In this ELT pipeline, the Extract and Load steps are already completed using Airbyte, with an additional Normalization step that transforms the resulting json blob fields to columns. This means that you can jump right into the Transformation step using dbt. There are two types of dbt tests:
- built-in tests: Built-in tests are the predefined generic tests that come with dbt Core and are usually defined as properties in your schema.yml file in the models folder. This is why they are often called schema tests.
- custom tests: Custom or bespoke tests are written in the tests folder and take the form of one-off SQL SELECT queries that validate the data based on returned values. Besides those two tests you can also implement tests from dbt packages, such as dbt-expectations and dbt-utils.
When writing dbt tests, it is important to define them in a meaningful and qualitative way based on the data and requirements. The value you get from testing is only as good as how well you define your tests.
Following, you will utilize both built-in and custom tests.
Built-in tests
In the schema.yml file of the models folder of your dbt project, you define properties using dbt’s built-in tests on data sources and models. dbt can run four different types of tests:
- Uniqueness: tests the column has unique values.
- Not null: tests the column does not have null values.
- Referential integrity: tests that the column values have an existing relationship with parent reference table.
- Accepted values: tests that the column contains only defined values as specified.
In your project, define a test on the data sources for uniqueness and not null on their respective hashID column.
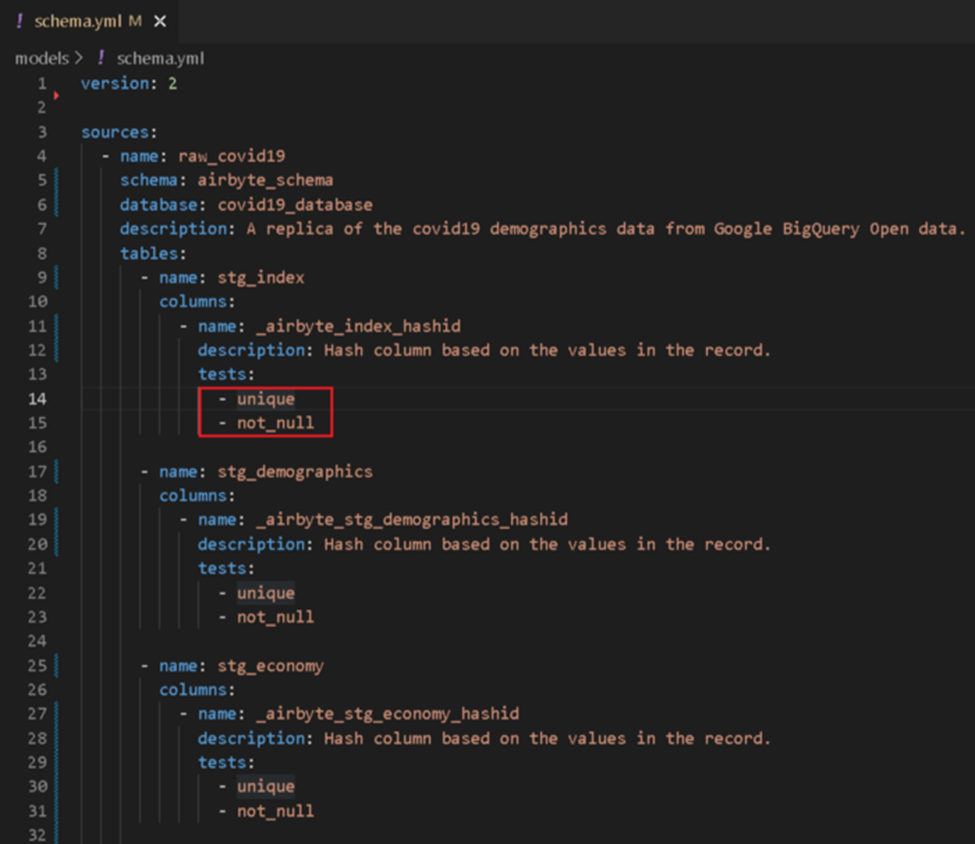
To test the specific test on the source table, use the following command: dbt test --select source:raw_covid19.stg_epidemiology
dbt test --select source:raw_covid19.stg_epidemiology
Using the dbt test command without any arguments will execute all the test cases and output a summary result. The result of a test can either be PASS, WARN, ERROR or SKIP. Below is the result of the test run for the specific data source:
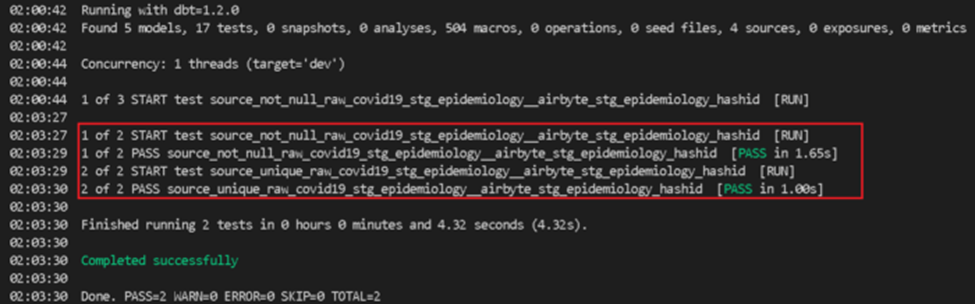
If the run leads to an error, dbt will output the error details. Below is a screenshot of running a test with an error:
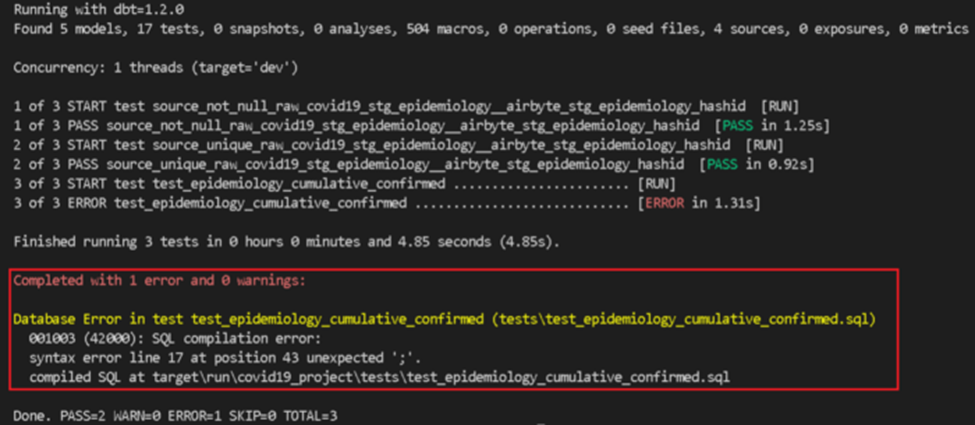
To test referential integrity, you use the field name relationships. The example below checks that for each location_key value in the base_epidemiology table, there exists another value under the location_key column in the base_index table.

For the accepted values test, you test that the location_key has only the specified values in the predefined range. Notice that the severity level has been configured to WARN. This means that instead of an error exception, it will display a warn with additional details.
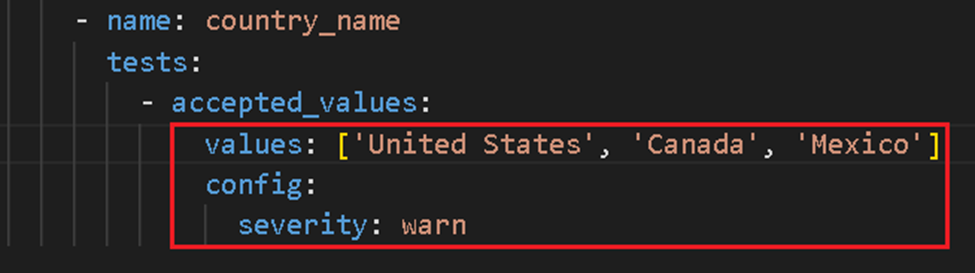
In the example above, if any of the values are not United States, Canada, or Mexico, dbt tests will give a WARN during test run. In the example below, 244 records did not match the accepted value test criteria:
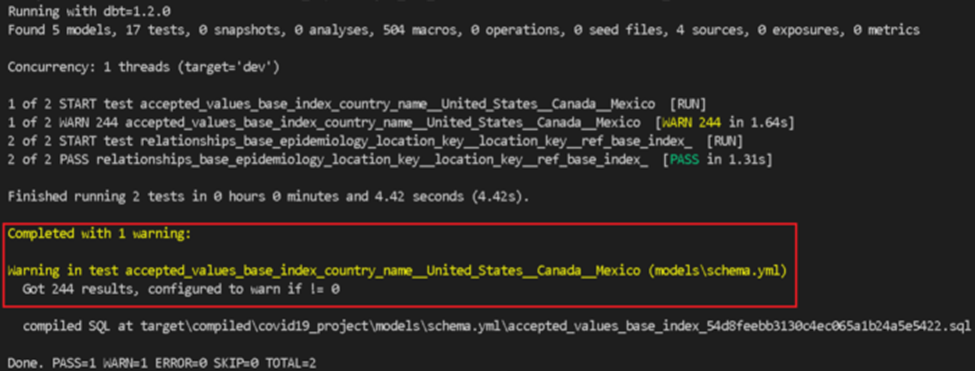
Custom tests
Custom tests are defined in the "tests" folder of your dbt project. They are written test assertions using dbt’s SQL SELECT statements. Custom tests pass if they don't return any data; otherwise the test outputs a non-passing error. You see this illustrated with three questions about the transformation that can be answered using custom tests:
1. Is every record from the epidemiology source table transformed into the model?
-- Test assertion for all records transformed. select location_key, date from {{ source('raw_covid19','stg_epidemiology' )}} except select location_key, date from {{ ref('covid19_model')}}
2. Is every value from the epidemiology confirmed cases greater than zero?
-- Test assertion for cumulative confirmed cases greater than 0. select location_key, date, sum(cumulative_confirmed) as total_cumulative_confirmed from {{ source('raw_covid19', 'stg_epidemiology' )}} group by location_key, date having not(total_cumulative_confirmed >= 0)
3. Is every value from the epidemiology cumulative cases greater than new cases?
-- Test assertion for cumulative confirmed greater than new confirmed cases select location_key, date, sum(new_confirmed) as total_new_confirmed, sum(cumulative_confirmed) as total_cumulative_confirmed from {{ ref('base_epidemiology')}} group by location_key, date having (total_new_confirmed > total_cumulative_confirmed)
To run only the dbt custom tests directly you can use the command with the selector argument:
dbt test --select test_type:singular
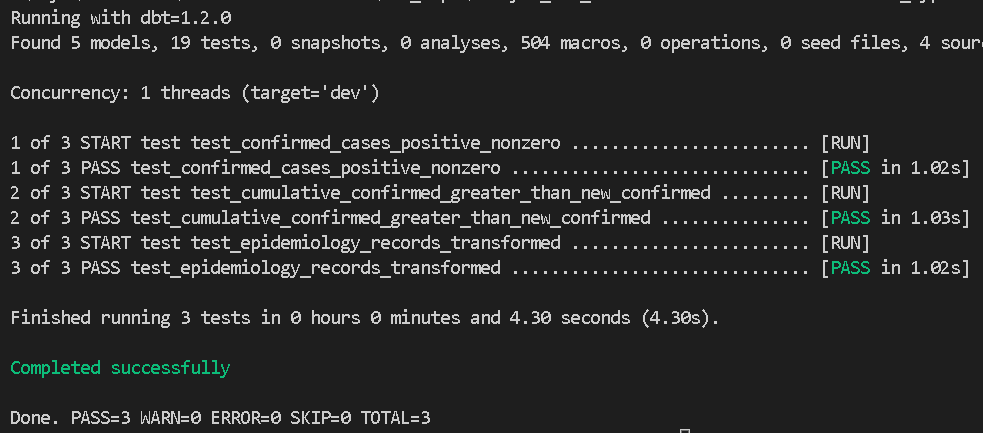
To illustrate a non-passing test, you can run the statement below to erase records from the dbt model table in the data warehouse:
-- Remove records from dbt model DELETE FROM COVID19_DATABASE.AIRBYTE_SCHEMA.COVID19_MODEL WHERE Date = '2021-03-23'; -- number of rows deleted: 5,522
Then re-run the custom tests, which should show FAIL for the particular test checking if all records transformed:
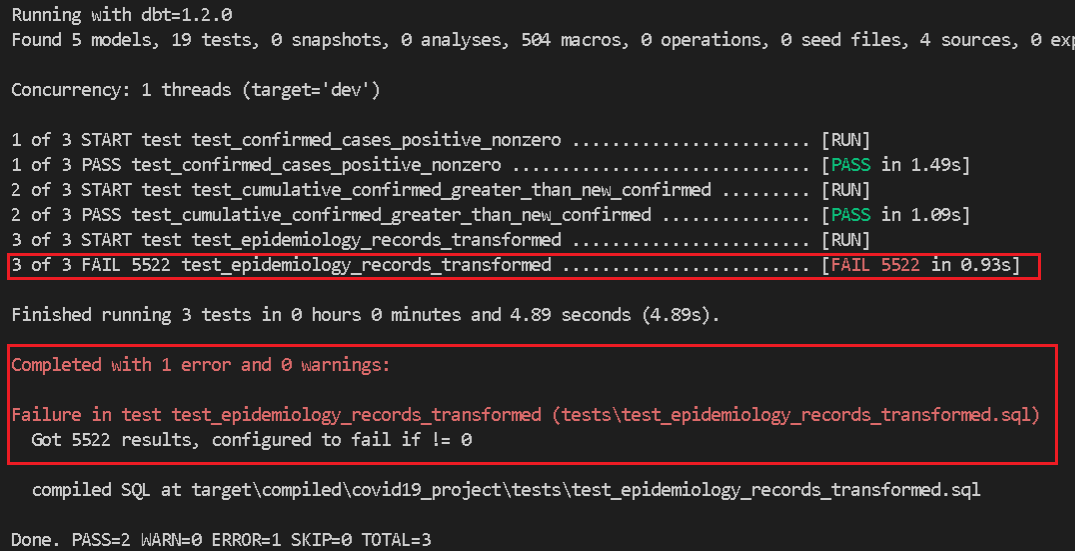
Custom tests are good for testing business transformations. In the dbt Lineage graph below, the tests defined are included with their dependencies. When executing the dbt test or dbt run commands without options the custom tests are also executed.
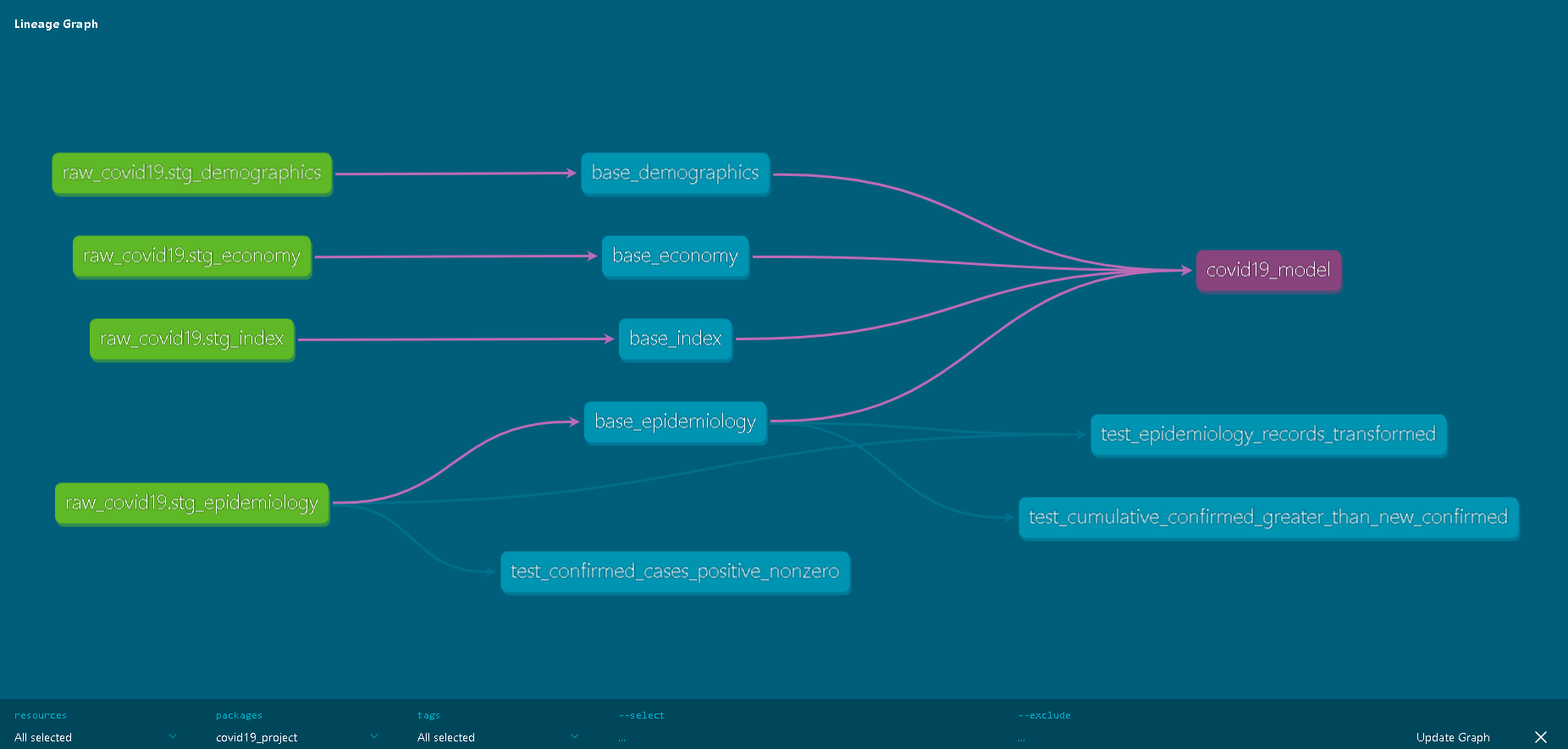
Conclusion
This wraps up the beginner’s guide to dbt testing. If you’re interested in digging deeper into dbt tests, read on here:
Monitoring
Schema change detection
Lineage monitoring