From Data Analyst To Founder | Bigeye Co-Founder Kyle Kirwan on Leadership in Tech
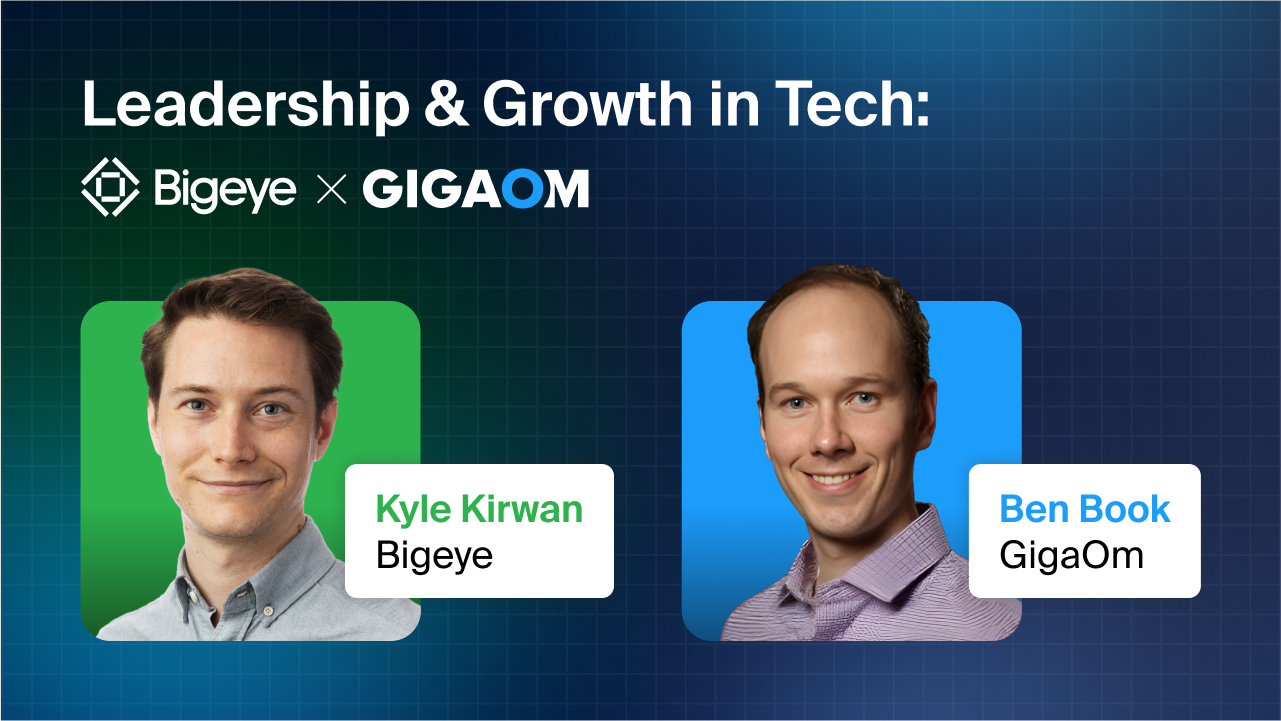
Get the Best of Data Leadership
Stay Informed
Get Data Insights Delivered
In this podcast episode, Kyle Kirwan, co-founder of Bigeye, walks Ben Book of GigaOm through his journey from a data analyst at Uber to launching a cutting-edge data observability company. With a background in industrial engineering, Kyle’s unconventional path gave him a fresh perspective on data science and product management, which has shaped how he navigates leadership in the tech space.
Kyle shares stories from his time at Uber, where he worked on scaling a global data platform. The lessons learned—managing data quality, lineage, and observability in a fast-growing enterprise—formed the foundation of Bigeye’s mission today.
Key takeaways:
- The power of data in driving strategic business decisions and innovation at scale.
- How to build trust in your data and make it central to high-impact areas of the business.
- Tackling scaling challenges by focusing on operational efficiency and data reliability.
- Why enterprises need data observability, particularly when balancing legacy systems with cloud technologies.
By the end, you’ll walk away with actionable insights into how data-driven leadership and smart decision-making can help enterprises scale their data systems and improve business outcomes.
Watch the full interview here:
Monitoring
Schema change detection
Lineage monitoring